JCTC: Physics-informed active learning for accelerating quantum chemical simulations
Recently, we published a paper in JCTC about the end-to-end physics-informed active learning with data-efficient construction of machine learning potentials. It shortens molecular simulation time to a couple of days which could have taken weeks of pure quantum chemical calculations.
The active learning is based on the physics-informed sampling of training points, which considers that the model can only make good predictions if it sees the data in the relevant regions. We assume that all required information should be available in the sampled PES points, that is energies while gradients provide more information that only allows somewhat better know the shape near the sampled points. Based on this, we develop a simple approach to judge how far we stray away from the sampled points by checking how much the main ML potential trained on energies and gradients deviates from the auxiliary model trained only on energies.
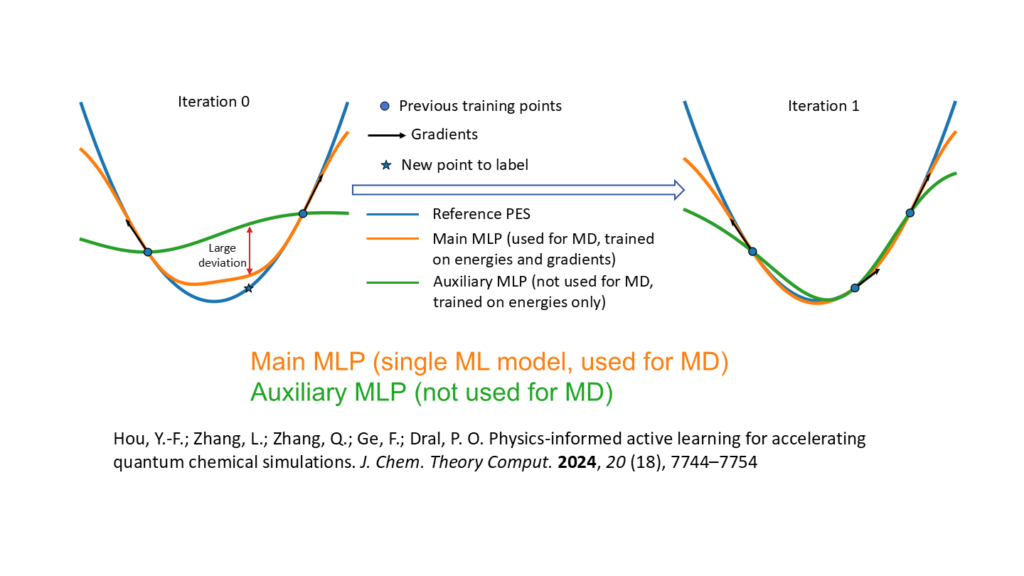
We also have automatic selection of initial data, based on the fact that adding more training points leads to small gains in accuracy after initial rapid improvement. It means that after some point, adding more points leads to diminishing returns and is not worth doing.
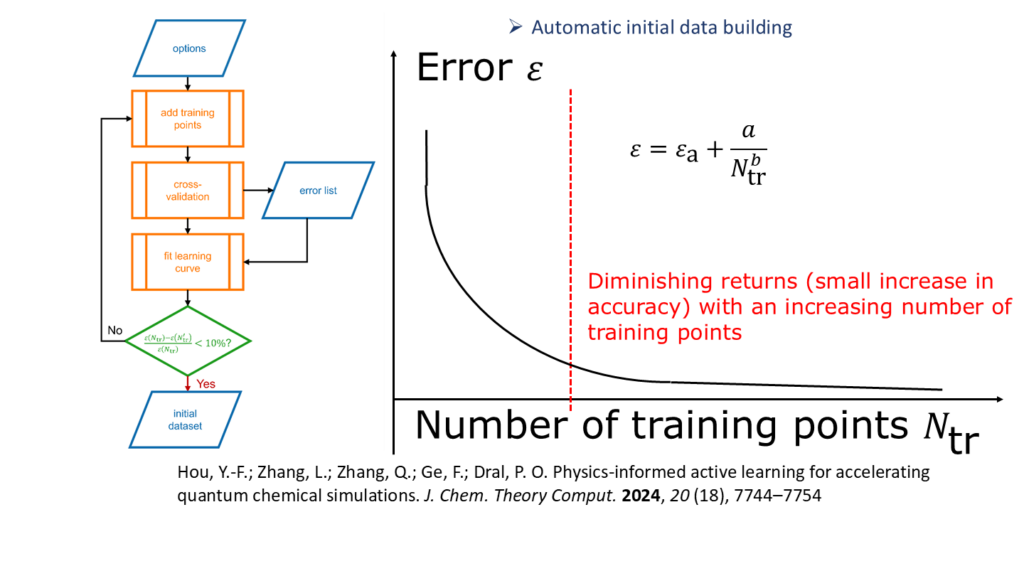
Choosing an uncertainty quantification threshold is also non-trivial, which we solve by choosing such a threshold that the predictions of 99% of the initial data set are confident – after all, we do not want that threshold to be so tight that the model is treated as uncertain even for lots of initial data. This, coupled with the automatic convergence monitoring allows us to build a fully automatic end-to-end protocol.
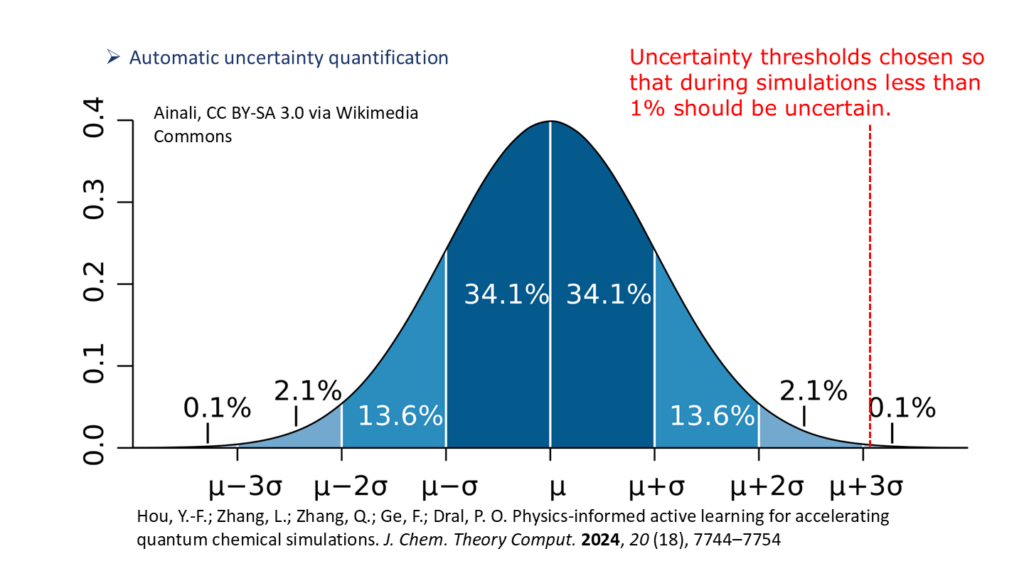
The versatility of this protocol is shown in the simulations of vibrational spectra, conformer search, and time-resolved mechanism of the Diels–Alder reaction. Our active learning protocol is available in MLatom and can be used as described in the online tutorial.
Please check out the hands-on online mini-course Modern AI and computational chemistry by Prof. Pavlo O. Dral.
Leave a Reply