Roundup of MLatom’s Year 2022. What to Expect in 2023?
MLatom team wishes you Happy Holidays and all success in New Year 2023!
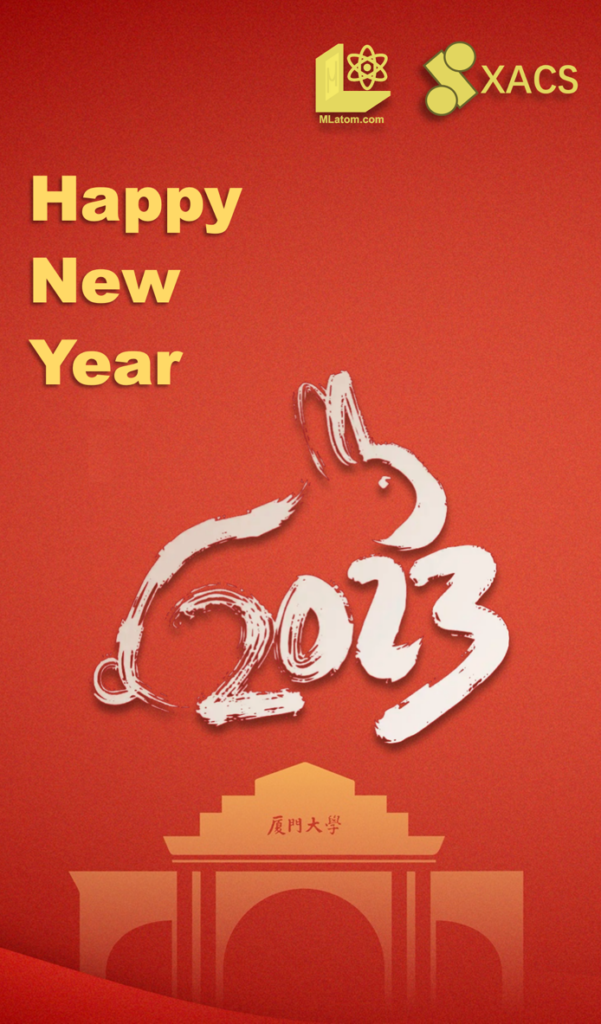
We use this occasion to bring to your attention our roundup of how MLatom became better in 2022 and provide a preview of what to expect in 2023.
In the cloud
MLatom joined XACS cloud computing as a co-founding member. This is by far the most exciting development as our users now can perform their desired atomistic machine learning simulations in the cloud, all for free and without the need to install all the software! You can try the calculations as described here. As a bonus, MLatom@XACS cloud computing allows performing simulations that are not even officially released in the MLatom package. Usually, such features are our latest developments for which we do not have a publication, so you get to test out something which is not even published! (or only published in a pre-print…). As yet another bonus, on the same cloud computing platform you can do other unique calculations not easily available elsewhere: valence bond theory and energy decomposition analysis provided by two other co-founding packages XMVB and XEDA.
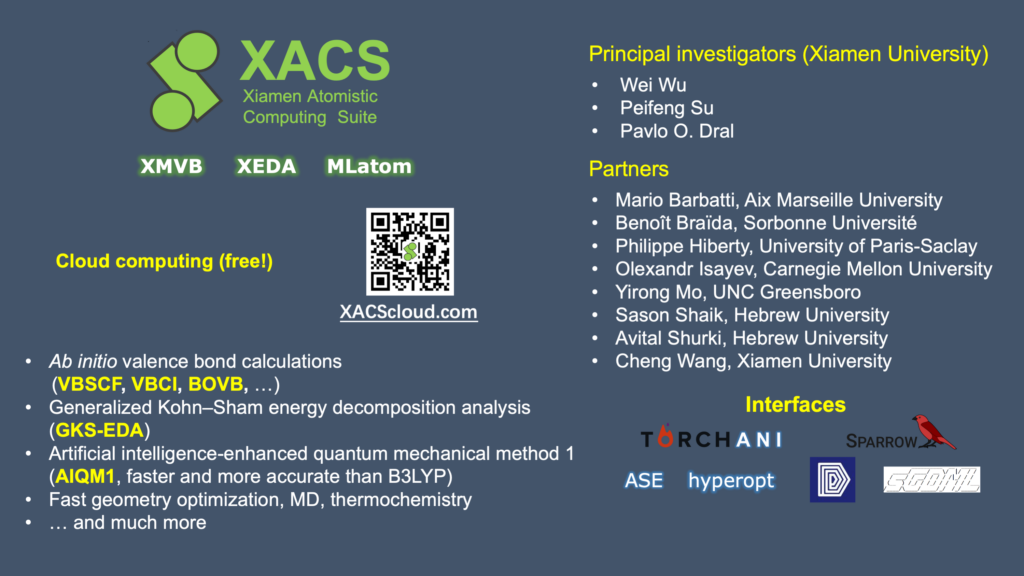
New manual
We have substantially upgraded (basically overwritten from scratch) all our manual to make it easier for you to find what you need to perform atomistic machine learning simulations. Here its bird-eye overview:
Simulations
- single-point calculations
- geometry optimizations (minima and transition states, IRC)
- frequencies & thermochemistry
- UV/vis spectra (ML-NEA)
- simulations with pre-trained models (AIQM1, ANI-1ccx, etc.)
- simulations with user-trained models
- NEW! two-photon absorption cross sections (ML-TPA)
- Only available on MLatom@XACS cloud:
- Coming soon:
- molecular dynamics
- IR spectra
Learning
- training popular ML models (KREG, ANI, sGDML, PhysNet, DPMD, GAP-SOAP, KRR-CM)
- training generic ML models (kernel ridge regression with many kernels)
- optimizing hyperparameters
- evaluating ML models (also with learning curves)
- Δ-learning
- self-correction
Data
- converting XYZ coordinates to molecular descriptor (RE, Coulomb matrix, …)
- analyzing data sets
- sampling (random, structure-based, farthest-point) and splitting datasets
We have also substantially increased the collection of our tutorials.
Better support and training
It is not just a much better manual. We have took our support to the next level. Not fun of an email and want an instant reply to a question about MLatom? Then use our Slack workspace! You can still drop an email to Pavlo if you prefer the old way.
In addition, we have started performing regular training of our users in different workshops. In 2022, we held our very first MLatom workshop as a part of the first XACS workshop, with lectures given by Pavlo and Olexandr Isayev (who is now also our partner in XACS). Then MLatom team taught how to use machine learning for simulations in the CECAM Machine Learning and Quantum Computing for Quantum Molecular Dynamics [MLQCDyn] school (see a tutorial with lectures and exercises).

New releases and features
We have released several upgrades mainly with new features but also with many technical improvements and bug fixes:
- accurate and extremely fast heats of formation with ANI-1ccx
- machine learning two-photon absorption (preprint)
- explicit learning of atomic forces by KREG and pKREG models which allow for creating very accurate internuclear potentials of molecules (preprint)
- more kernel functions for kernel ridge regression: periodic kernel (keywords:
kernel=Gaussian periodKernel period=R
, where R is a user-defined period) and decaying periodic kernel (keywords:kernel=Gaussian decayKernel period=R sigmap=S
, where R is a user-defined period and S is a user-defined length scale for a periodic part). Other keywords are the same as for the KRR with the Gaussian kernel function
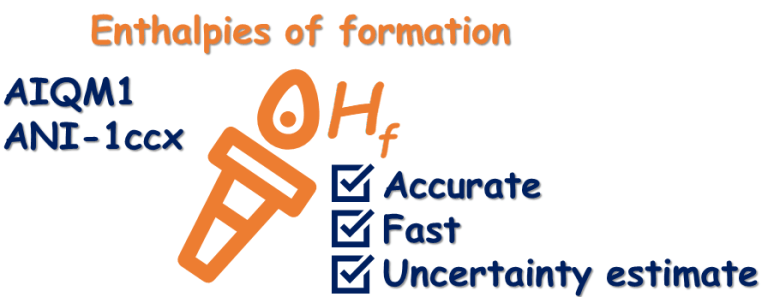
New research and publications by MLatom team
We are researchers and not a company. Our bread and butter is doing research with MLatom. This year’s overview of our new research and educational material just shows very small part of what MLatom is capable of:
- Book where for many tutorials MLatom is used: Quantum Chemistry in the Age of Machine Learning, Pavlo O. Dral, Ed. Elsevier: 2023. ISBN: 9780323900492. Companion website to the book with complementary materials, data, codes, etc. (blog post)
- Interpretable Machine Learning of Two-Photon Absorption. accepted. Preprint on ChemRxiv.
- A comparative study of different machine learning methods for dissipative quantum dynamics. Mach. Learn. Sci. Technol. 2022, 3, 045016. (blog post)
- The Newton-X platform: new software developments for surface hopping and nuclear ensembles. J. Chem. Theory Comput. 2022, 18, 6851–6865. (blog post)
- Machine Learning for Designing Mixed Metal Halides for Efficient Ammonia Separation and Storage. J. Phys. Chem. C, 2022, 126, 12184–12196. DOI: 10.1021/acs.jpcc.2c02586. (blog post)
- One-shot trajectory learning of open quantum systems dynamics. J. Phys. Chem. Lett. 2022, 13, 6037–6041. (blog post)
- Toward Chemical Accuracy in Predicting Enthalpies of Formation with General-Purpose Data-Driven Methods. J. Phys. Chem. Lett. 2022, 13, 3479–3491. (blog post | tutorial with MLatom calculations)
- Predicting the future of excitation energy transfer in light-harvesting complex with artificial intelligence-based quantum dynamics. Nat. Commun. 2022, 13, 1930. DOI: 10.1038/s41467-022-29621-w. (blog post)
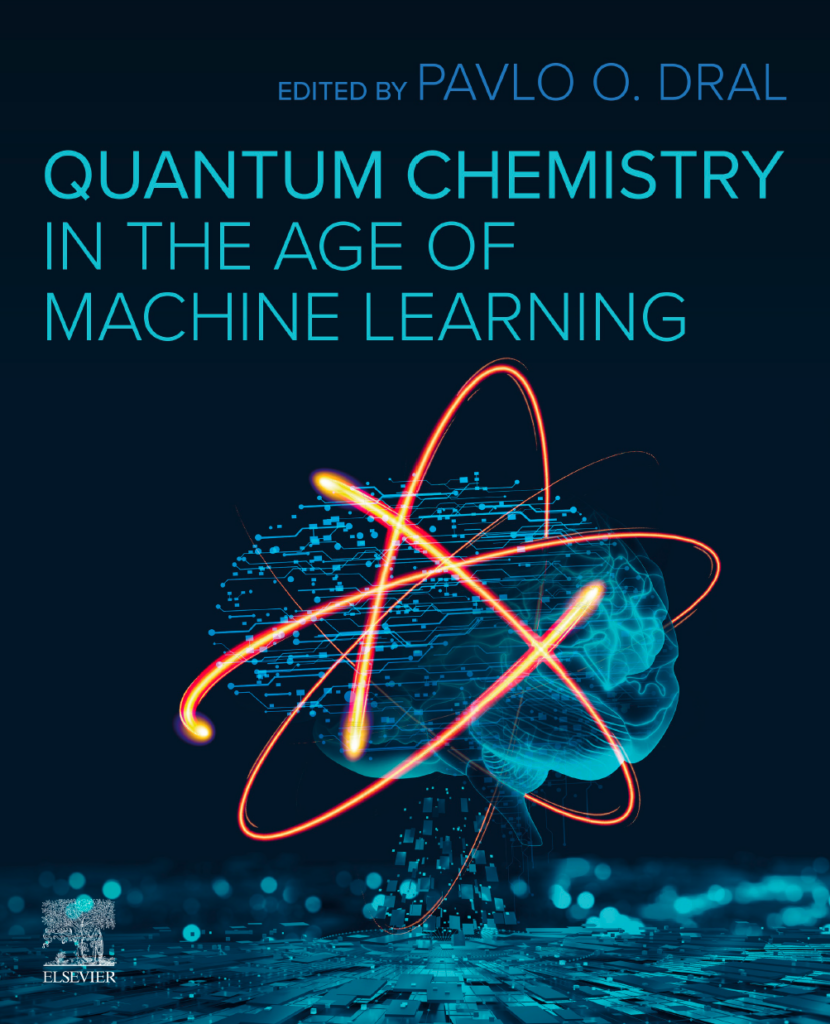
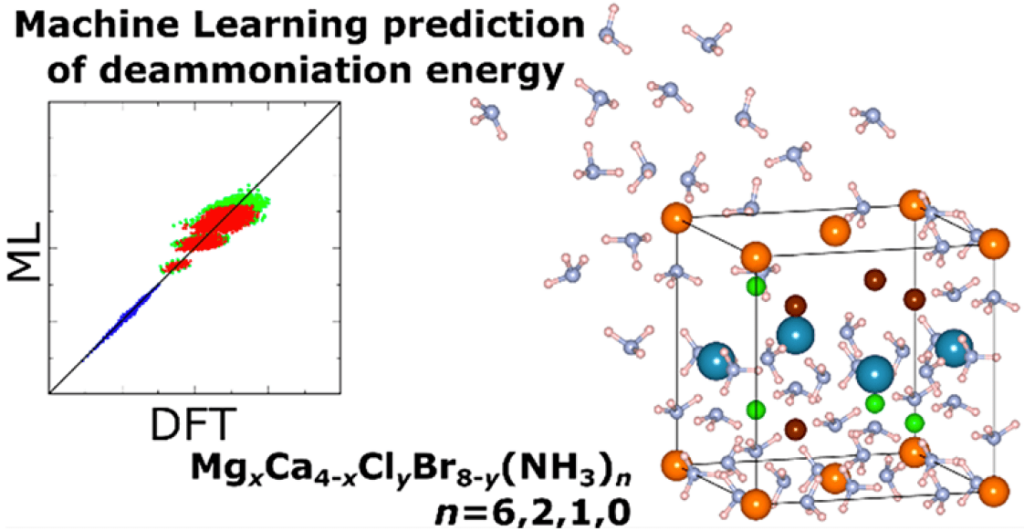
What’s next in 2023?
In 2023 we plan to upgrade all aspects of MLatom to make it even easier to use and to provide more and better features. Some new features that are going to be released in 2023 are:
- quantum dynamics with machine learning (already available in MLatom@XACS but not yet released in MLatom package) via interface to Arif Ullah’s MLQD program supporting our AI-QD, OSTL, and recursive quantum dynamics with machine learning
- make AIQM1 even easier available via releasing our interface to SCINE Sparrow (whose development version already contains AIQM1 in itself, see our preprint).
- release implementations of molecular dynamics and infrared spectra simulations implemented by Yi-Fan Hou.
- release our implementation of 4D-spacetime atomistic artificial intelligence models (an absolutely new way of doing molecular dynamics and beyond, see our preprint).
- last but not least, we finally plan to release MLatom as a ‘true’ Python package so that it can be used as a module for your own workflows in your Jupyter notebooks and beyond.
We are always looking forward to your suggestions on how to improve the user experience with MLatom.
We wish you a Happy New Year 2023!
Sincerely yours,
Pavlo O. Dral
MLatom founder and main developer
Leave a Reply